Automatic pollen recognition – developments and perspectives
Automatic pollen recognition has been developed based on socalled gray-scale invariants, which characterise pollen grains independently from their position and orientation on the microscopic sample. Thus, pollen features can be extracted from the gray-scale images of transmitted light and fluorescence microscopy. In a first step, this approach is demonstrated with Ambrosia pollen of samples from a Burkard sampler, where pollen are collected from ambient air on a sticky tape mounted on a slowly rotating drum. Self-learning Support Vector Machines create a classification model from the gray-scale invariants of the particles on three Burkard samples from Mezzana (Ticino), Switzerland. Automatic pattern recognition is tested with 13 other samples from the period between July, 20th and September, 9th 2004. A recall of 77.3 % has been found for the automatic recognition of Ambrosia pollen, together with a precision of 84.0 % for this classification. Falsely negative classified objects can partly be ascribed to agglomerated pollen, the number of falsely positive classified objects can be reduced by a more specific classification mode. Automatic pollen recognition provides the basis for the development of a fully automated system that combines sampling, particle deposition onto a surface suitable for optical analysis, automatic preparation, microscopic imaging techniques, pattern recognition and the hourly output of number concentration of airborne pollen.
Vorschau
Zitieren
Zugriffsstatistik
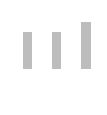