Establishment of a time-sensitive crop database of Germany based on multi-temporal Sentinel-1 and Sentinel-2 Data
One of the aims of the Common Agricultural Policy (CAP) is ensuring that farmers within the European Union (EU) make a reasonable living through the provision of subsidies. This serves as a great incentive to the farmers as they ensure that consumers have a stable supply of food. In order to ensure that these subsidies are judiciously expended, at the various country levels, computer-aided interpretation of very high resolution imagery and field campaigns are used to check the aerial claims of farmers before the subsidies are given out. These validated claims are consolidated into the Land Parcel Identification System (LPIS). Even though the LPIS is becoming freely available in a number of EU countries, there is still restricted access to this data in many regions of Germany. Even where they are available, the dataset is published annually and sometimes outdated. This makes it difficult to identify crop types at any point in time before the release of the dataset. This research seeks to tackle these issues by making use of the freely available Sentinel-1 and Sentinel-2 data and existing LPIS dataset of Germany for 2016 and 2017.This research adopts a two-step OBIA workflow that consists of parcel-identification and crop type mapping. In the first step, various features including vegetation indexes from Sentinel-2 and radar polarization ratios from Sentinel-1 are calculated. The optimal shape, scale and compactness parameters for delineating agricultural parcels using the Multiresolution Segmentation (MRS) algorithm for every region of Germany is identified based on the extracted features. The parameter combinations whose segmentation result has the highest geometric match to the LPIS parcels are considered the optimal ones. In the process, the optimal features are also identified. In the second step, using the LPIS parcels of 2017 as the reference layer, the crop type of newly segmented agricultural parcels, regardless of which point in time and geolocation, would be identified using a Transfer Learning (TL) scheme, which is still being developed. Initial results from the first step show that Sentinel-1 is not suited for identifying agricultural parcels largely due to the speckled and non-homogeneous nature of the images even after applying a multi-temporal speckle filter. With respect to the mono-temporal Sentinel-2 datasets, the scenes acquired during the summer season have the highest geometric match to the LPIS parcels. However, the highest geometric match was only realized by using a multi-temporal dataset that covered five different dates of the 2017 growing season.
Dateien
Zitieren
Zugriffsstatistik
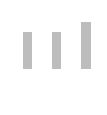
Rechte
Nutzung und Vervielfältigung:
Alle Rechte vorbehalten