Recursive feature elimination in random forest classification supports nanomaterial grouping
Nanomaterials (NMs) can be produced in numerous different variants of the same chemical substance. An in-depth safety assessment for each variant by generating test data will simply not be feasible. Thus, NM grouping approaches that would significantly reduce the time and amount of testing for novel NMs are urgently needed. However, identifying structurally similar NM variants remains challenging as many physico-chemical properties could be relevant. Here, we aimed at emphasizing on the value of machine learning models in the process of NM grouping by considering a case study on eleven selected, well-characterized NMs. To that end, we linked physico-chemical properties of these NMs to characterized hallmarks for inhalation toxicity. We applied unsupervised and supervised machine learning techniques to determine which combination of properties is most predictive. First, we assessed NM similarity in an unsupervised manner using principal component analysis (PCA) followed by subsequent superposition of activity labels combined with a k-nearest neighbors approach. Then, we used random forests (RFs) as a supervised machine learning technique which directly uses the knowledge on the activity class in the process of defining NM similarity. Thus, similarity was defined only on those properties showing the highest correlation with the activity and therefore had the highest discriminative power. In order to improve the performance, we then used recursive feature elimination (RFE) to delete uninformative features biasing the results. The best performance was achieved by the reduced RF model based on RFE where a balanced accuracy of 0.82 was obtained. Out of eleven different properties we determined zeta potential, redox potential and dissolution rate to have the strongest predicting impact on biological NM activity in the present dataset. Though the dataset is too small with respect to the number of NMs studied and the applicability domain is expected to be very limited due to the fact that only few material classes were covered, our study demonstrates how machine learning and feature selection methods can be implemented for identifying the most relevant physico-chemical NM properties with respect to toxicity. We suggest that once the most relevant properties have been detected in a model built on a sufficient number of different NMs and across multiple NM classes, they should obtain special emphasis in future grouping approaches.
Vorschau
Zitieren
Zugriffsstatistik
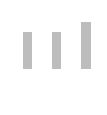