How to determine temporal yield variances of various cropping systems for modelling farmers’ production risk – Illustrated by results from a long-term field trial
When making choices among different cropping systems like conventional farming, organic farming or cropping systems without pesticides, but with mineral fertilizers, risk averse farmers will not only account for expected (i.e. average) farm income but also for income stability. The smaller the variance of a farm’s total contribution margin, the more stable is the income from a cropping system. The overall income variance can be calculated and included in quadratic risk programming models that apply the expected value-variance criterion when temporal (co-)variances of the contribution margins of the single crops are known. In this context, there is a lack in empirically well-founded approaches to identify the latter. Thus, taking a single farmer’s perspective, we first outline a way to derive temporal (co-)variances of contribution margins for crops grown at one location. Second, neglecting variances of crop prices and variable costs, we show for different cropping systems how to consistently estimate temporal crop yield (co-)variances based on available yield data from a long-term field trial by the Julius Kühn-Institut in Dahnsdorf (Germany). The four cropping systems were (b1) without fertilizer and pesticides; (b2) without fertilizer but with pesticides; (b3) with fertilizer but without pesticides; and (b4) with fertilizer and pesticides. For each system, we estimated a covariance matrix for yield data of winter rye, winter barley and pea grown from 1998 to 2021 using a mixed effects model. Moreover, we calculated means, standard deviations and coefficients of variation for the different crop yields. The size of the (co-)variances found is a valuable indicator for their order of magnitude to be used as input in quadratic risk programming with the intention of optimizing a cultivation program that accounts for individual farmers’ preferred levels of risk. Further, our statistical approach should be applied to other long-term field experiments to get deeper insights into average yield level and (co-)variance structures of crops grown in different cropping systems.
Dateien
Zitieren
Zugriffsstatistik
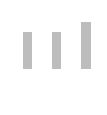
Rechte
Rechteinhaber: 2023 Elsevier B.V.
Nutzung und Vervielfältigung:
Alle Rechte vorbehalten