Grassland mowing event detection using combined optical, SAR, and weather time series
The European Union’s Common Agricultural Policy (CAP) and the Habitats Directive aim to improve biodiversity in agricultural landscapes. Both policies require enormous monitoring, which can be facilitated by remote sensing. Use intensity, measured by mowing frequency is an important indicator of biodiversity in permanent grasslands. The frequency and timing of mowing can be determined using satellite remote sensing because photosynthetically active biomass changes rapidly in response to mowing. However, the rapid regrowth of grasses requires very dense satellite time series for reliable detection. Radar time series can complement optical time series and fill in cloud-related gaps to overcome this problem. Additional weather data can support the detection of grassland mowing events, as mowing events are associated with specific meteorological conditions. However, previous studies have not fully exploited both potentials or different machine learning approaches for mowing event detection. This study presents a new transferable two-step approach to detect grassland mowing events using combined optical and SAR data and additional weather data. First, we filled cloud-related gaps in optical time series using a supervised machine learning regression with optical and SAR data. We then classified time series sequences of optical, SAR and weather data into mown and unmown using four different machine learning algorithms. We used time series of NDVI and EVI (combined Sentinel-2 and Landsat 8), SAR backscatter, six-day interferometric coherence, backscatter radar vegetation index, backscatter cross-ratio (Sentinel-1), and temperature and precipitation sums. Our test sites are distributed across Germany and cover the entire gradient of grassland use intensities. Mowing events could be detected with F1 values of up to 89%, first cut with up to 94%. Our results show no structural advantage of infilling time series with machine learning over linearly interpolated time series. The combined Sentinel-2 and Landsat-8 time series provided dense time series with mostly median gaps less than 20 days, which proved sufficient to reliably detect mowing events. SAR data were not essential for mowing event detection in our study, but weather data improved classification results for models trained on all areas and years. However, when the model was transferred to unknown years or areas that were not used for training, SAR data improved detection accuracy, whereas weather data degrade it. Models trained on all years but not all study sites detected mowing events with an accuracy of up to F1 = 76%. Models trained with all regions but not all years detected mowing events in untrained years with F1 up to 80%.
Vorschau
Zitieren
Zugriffsstatistik
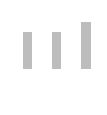