Optimized bare soil compositing for soil organic carbon prediction of topsoil croplands in Bavaria using Landsat
Soil Organic Carbon (SOC) is amongst others an indicator for soil degradation and soil health of croplands. Induced by recent policy initiatives, awareness for high resolution SOC maps and techniques to estimate changes is increasing. For area-wide mapping approaches with at least a field resolution, Earth Observation is a valuable data source to extract bare soil areas and to quantify SOC contents for these areas. In this context, compositing techniques of multi-temporal image archives are widely used to overcome the limitation of vegetation cover of fields during the overpass of the satellite. Comparing current bare soil compositing approaches, two aspects are of particular importance: 1) the index for bare soil selection and 2) the length of the compositing period for recurrent analyses. In this study, we applied the Soil Composite Mapping Processor (SCMaP) to the full archive of Landsat data between 2005 and 2019 to optimize parameters for soil reflectance composite (SRC) generation of multitemporal satellite imagery for SOC predictions. For this purpose, three spectral indices (PV + BLUE, PV + IR2, and NBR2) for SRC generation were implemented in the SCMaP chain. For all three indices a validation of the extracted bare soil dates with field observations and phenological information from the crop calendar showed a reliable extraction of bare soil dates. Due to the crops in the investigation area, spring and autumn months indicated the highest proportion of correctly selected bare soil dates. We also analyzed the SOC modeling capabilities of different composed SRCs (indices and varying seasonal and temporal lengths) in combination with available legacy data. In comparison to a seasonal pre-selection of scenes (spring and autumn months) included in the SRC, the different indices showed a minor influence on SOC modeling. However, PV + BLUE performed best (R2: 0.56 – 0.72, RMSE: 1.09 – 1.29%, RPD: 1.51 – 1.91). Furthermore, we compared the SOC model capabilities for different SRC compositing lengths (3-, 5-, 7-, 10- and 15-years). For PV + BLUE and PV + IR2, longer compositing lengths (from three to 15 years) resulted in an increase of the model accuracies and performances. However, for NBR2 this was not as clear. Based on the results at least a 5-year compositing period is required for recurrent SOC predictions using Landsat data.
Dateien
Zitieren
Zugriffsstatistik
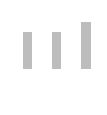
Rechte
Rechteinhaber: 2023 International Society for Photogrammetry and Remote Sensing, Inc. (ISPRS). Published by Elsevier B.V.
Nutzung und Vervielfältigung:
Alle Rechte vorbehalten