How can we justify grouping of nanoforms for hazard assessment? Concepts and tools to quantify similarity
The risk of each nanoform (NF) of the same substance cannot be assumed to be the same, as they may vary in their physicochemical characteristics, exposure and hazard. However, neither can we justify a need for more animal testing and resources to test every NF individually. To reduce the need to test all NFs, (regulatory) information requirements may be fulfilled by grouping approaches. For such grouping to be acceptable, it is important to demonstrate similarities in physicochemical properties, toxicokinetic behaviour, and (eco)toxicological behaviour.
The GRACIOUS Framework supports the grouping of NFs, by identifying suitable grouping hypotheses that describe the key similarities between different NFs. The Framework then supports the user to gather the evidence required to test these hypotheses and to subsequently assess the similarity of the NFs within the proposed group.
The evidence needed to support a hypothesis is gathered by an Integrated Approach to Testing and Assessment (IATA), designed as decision trees constructed of decision nodes. Each decision node asks the questions and provides the methods needed to obtain the most relevant information. This White paper outlines existing and novel methods to assess similarity of the data generated for each decision node, either via a pairwise analysis conducted property-by-property, or by assessing multiple decision nodes simultaneously via a multidimensional analysis.
For the pairwise comparison conducted property-by-property we included in this White paper:
• A Bayesian model assessment which compares two sets of values using nested sampling. This approach is new in NF grouping.
• A Arsinh-Ordered Weighted Average model (Arsinh-OWA) which applies the arsinh transformation to the distance between two NFs, and then rescales the result to the arsinh of a biologically relevant threshold before grouping using OWA based distance. This approach is new in NF grouping.
• An x-fold comparison as used in the ECETOC NanoApp.
• Euclidean distance, which is a highly established distance metric.
The x-fold, Bayesian and Arsinh-OWA distance algorithms performed comparably in the scoring of similarity between NF pairs. The Euclidean distance was also useful, but only with proper data transformation. The x-foldmethod does not standardize data, and thus produces skewed histograms, but has the advantage that it can be implemented without programming knowhow.
A range of multidimensional evaluations, using for example dendrogram clustering approaches, were also investigated. Multidimensional distance metrics were demonstrated to be difficult to use in a regulatory context, but from a scientific perspective were found to offer unexpected insights into the overall similarity of very different materials.
In conclusion, for regulatory purposes, a property-by-property evaluation of the data matrix is recommended to substantiate grouping, while the multidimensional approaches are considered to be tools of discovery rather than regulatory methods.
Vorschau
Zitieren
Zugriffsstatistik
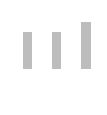