Combination of an Automated 3D Field Phenotyping Workflow and Predictive Modelling for High-Throughput and Non-Invasive Phenotyping of Grape Bunches
In grapevine breeding, loose grape bunch architecture is one of the most important selection traits, contributing to an increased resilience towards Botrytis bunch rot. Grape bunch architecture is mainly influenced by the berry number, berry size, the total berry volume, and bunch width and length. For an objective, precise, and high-throughput assessment of these architectural traits, the 3D imaging sensor Artec® Spider was applied to gather dense point clouds of the visible side of grape bunches directly in the field. Data acquisition in the field is much faster and non-destructive in comparison to lab applications but results in incomplete point clouds and, thus, mostly incomplete phenotypic values. Therefore, lab scans of whole bunches (360) were used as ground truth. We observed strong correlations between field and lab data but also shifts in mean and max values, especially for the berry number and total berry volume. For this reason, the present study is focused on the training and validation of dierent predictive regression models using 3D data from approximately 2000 dierent grape bunches in order to predict incomplete bunch traits from field data. Modeling concepts included simple linear regression and machine learning-based approaches. The support vector machine was the best and most robust regression model, predicting the phenotypic traits with an R2 of 0.70–0.91. As a breeding orientated proof-of-concept, we additionally performed a Quantitative Trait Loci (QTL)-analysis with both the field modeled and lab data. All types of data resulted in joint QTL regions, indicating that this innovative, fast, and non-destructive phenotyping method is also applicable for molecular marker development and grapevine breeding research.
Vorschau
Zitieren
Zugriffsstatistik
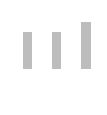