Multi-model ensemble improved the prediction of trends in soil organic carbon stocks in German croplands
Various soil organic carbon (SOC) models and additional methods to calculate carbon (C) input to soil are used to simulate SOC stocks, e.g. for national greenhouse gas inventories. Most of the SOC models used on a regional to national scale share the principle of process-based multi-compartment models, but differ in their initialization or decomposition modifying factors. We performed a model comparison to identify the model or multi-model ensemble that best fits trends in SOC stocks of German croplands. We combined six SOC models (C-TOOL, CCB, CENTURY, ROTH-C, ICBM and YASSO07) with five C input estimation methods (bolinder, bze, ccb, ctool, ipcc-nir), producing a model set of 30 model combinations. We used data from agricultural permanent soil monitoring sites in Germany to compare modeled and measured trends of SOC stock time series. The absolute mean error (AME) of the trends for the model combinations varied between 0.01 Mg ha−1 a−1 and 0.86 Mg ha−1 a−1, with C-TOOL, CENTURY and YASSO07 generally showing smaller AME than ICBM and CCB. The scatter of the modeled trends of the 30 model combinations indicated that the choice of SOC model was more important than the choice of C input estimation method. A multi-objective criterion was used to summarize results from AME, root mean squared error (RMSE) and the probability that the measured trend lies within the confidence interval of the modeled trend. We set up a multi-model ensemble consisting of all 30 model combinations, and reduced the number of participating models until the multi-objective criterion of the ensemble was smallest. A multi-model ensemble with nine model combinations was found to be the best ensemble. The multi-model ensemble performed better than the single model combinations, with AME of 0.004 Mg ha−1 a−1 and RMSE of 0.56 Mg ha−1 a−1. Based on our results, we recommend the use of a multi-model ensemble for predicting trends in SOC stocks as it reduces structural uncertainties and solves the problem of choosing the right SOC model or C input estimation method.
Dateien
Zitieren
Zugriffsstatistik
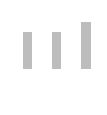
Rechte
Nutzung und Vervielfältigung:
Alle Rechte vorbehalten