Improving imputation quality in BEAGLE for crop and livestock data : [Preprint]
Imputation is one of the key steps in the preprocessing and quality control protocol of any genetic study. Most imputation algorithms were originally developed for the use in human genetics and thus are optimized for a high level of genetic diversity. As the software BEAGLE offers the user considerable flexibility to tune the algorithm to the specific genetic structure of the respective dataset. Different versions of BEAGLE were evaluated on genetic datasets of doubled haploids of two European landraces in maize, a commercial breeding line and a diversity panel in chicken, respectively, with different levels of genetic diversity and structure. BEAGLE 5.0 showed the best performance and was less dependent on adapted parameter settings than the earlier versions. For all versions, the parameter of the effective population size had a major effects on the error rate for imputation of ungenotyped markers, reducing error rates by up to 98.5%. For BEAGLE 4.0 and 4.1 imputation accuracies were further improved by tuning parameters like modelscale, buildwindow and nsamples. The number of markers with extremely high error rates for the maize datasets were more than halved by the usage of a flint reference genome (F7, PE0075 etc.) instead of the commonly used B73. On average, error rates for imputation of ungenotyped markers were reduced by 8.5% by excluding genetically distant individuals from the reference panel. Strategies to find a balance between representing as much of the genetic diversity as possible while avoiding the introduction of noise by including genetically distant individuals are discussed.
Nachfolger
Dateien
Zitieren
Zugriffsstatistik
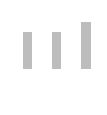
Rechte
Nutzung und Vervielfältigung:
Alle Rechte vorbehalten